Recognizing name activities
35 Fun Name Activities Perfect For Preschoolers
You are here: Home / Activities / Learning / Literacy & ABCs / 35 Nifty Name Activities Perfect For Preschoolers
21 Jul
Literacy & ABCs
PopularPreschoolersName
Resources
Spelling53 Comments
SHARE POST
Get your child excited about writing with 35 fun name activities that are perfect for preschoolers to work on recognition and spelling!
Last year in three-year-old preschool, names were a big deal. So I can only suppose they will be again this year in four-year-old preschool.
Henry learned to recognize his name pretty early on.
At first, he thought any word that started with (or even had) an H meant it was his name. But he soon learned that there was more to it than that.
Throughout the year they worked on name recognition and spelling. By the end of last year, Henry was able to spell his first name (and write it!!).
He even was able to spell it backward, which shocked me!
Now, it’s onto our last name… which for some reason seems daunting to me.
35 Fun Name Activities Perfect For Preschoolers
But with some help of these activities I found, it should come fast enough. Just applying it to our last name instead of his first!
Have some fun with your kids learning to recognize their very own name (it’s very special to them, make it be a special thing!) and then learn to spell it too! They’ll be so proud and you’ll be one proud parent!
Get the FREE Learn Your Name Download
Here are 35 name activities for your preschooler learn to recognize and spell their name!
In case you missed earlier this week, we covered the ABCs and Numbers!
Name Activities for Recognition
Put their name up everywhere. Label everything of theirs with their name. Use their name in your activities.
Have fun with it!
- Squirt their name! Create a fizzing reaction using baking soda sidewalk paint and vinegar!
- Write their name first and have them trace it with pipe cleaners in an activity from Parents.
com!
- Use candles to write their name, with an idea from Fairy Dust Teaching Kindergarten, and watch as the magic happens when they paint over it with watercolors!
- Make homemade playing cards with photos and names of your family, just like Teach Mama.
- Similarly, Busy Kids = Happy Mom creates a “Who Am I” book to label important people (and your child!) with their names.
- Create a pom pom name craft, like Learning 4 Kids.
- Write out their name with food. Play Dr. Mom uses “ants on a log.“
- Use these color by number name printables from Royal Baloo to have your child color their name!
- Try a sensory bin letter hunt, from Fun Learning for Kids, to recognize letters that are in their name.
- Spell your child’s name, then compare how long it is to other names, with an idea from One Sharp Bunch based on the book Chrysanthemum by Kevin Henkes.
- Teach Preschool shows how to collage their name with paper scraps onto their prewritten name.
Display this name recognition activity on their door!
- Write their name in glue and color over them to make prints, like Excellence in Early Childhood Education!
- Make your own DIY name recognition kit with this activity idea from Fun-A-Day.
- Use clothespins to make a simple name recognition matching game, from School Time Snippets.
Name Recognition Activities
Fizzy Sidewalk Paint
Hands On As We Grow
Name Recognition Plate Game
Little Giraffes
Pipe Cleaner Name
Parents
Secret Message Name Writing
Fair Dust Teaching
Family Name Playing Cards
Teach Mama
DIY Name Book
Busy Kids, Happy Mom
Crayon Resist Watercolor Name Placemats
Laura's Lily Pad
Ants on a Name Log
Play Dr. Mom
Color By Number Names
Royal, Baloo & Logi-Bear, too!
Sensory Name Recognition Tray
Smiling Like Sunshine
Comparing Name Length
One Sharp Bunch
Name Collage
Teach Preschool
Glue Traced Name Rubbings
Excellence in Early Childhood Education
DIY Name Kit
Fun-A-Day
Clothespin Name Recognition Activity
School Time Snippets
Get the FREE Learn Your Name Download
Name Activities for Spelling
Once they can recognize their name easily, spelling it in an activity comes easily too.
If they’ve seen it written out a million times, they’re likely to remember how it looks and what order it comes in.
- Use the computer and have them type their name using the keyboard.
- String up letters in their name around the room and have them go on a hunt!
- The Imagination Tree spells out names using play dough letter impressions. Here are my favorite tips on how to make homemade play dough.
- Danya Banya bakes and spells their name with letter shaped cookies!
- Make name rockets like Ms. Solano’s Kindergarten!
- Tip Junkie uses a paint stick and clothespins with letters of their name.
- The Busy Toddler likes to use Post-it Notes for a fun name spelling activity!
- Make necklaces with letter beads like the Little Giraffes!
- Make a name cube, roll it and spell your name with a game from Toddler Approved.
- Unscramble the letters to spell your name, as seen on Kids Activities Blog.
- Hunt for letters of their name on a walk with an idea from NurtureStore.
- Label rocks with letters of their name and use it to spell, just like Time for Play.
- Fish for letters of their name, like Fun Learning for Kids, and spell it out!
- Frugal Fun 4 Boys & Girls like to stamp their names with paint.
- Create a name mobile, as seen on Cere’s Childcare! Stamp or print the letters and have your child string them up in order!
- Shoot for the letters of your name in order with a cork! Some archery fun from Toddler Approved.
- Make your own customized handwriting worksheets on HandWritingWorksheets.com.
- Happy Hooligans makes these easy name puzzles for your child to put together!
- Having Fun At Home uses ABC blocks to spell their name in this activity. Though there’s usually a catch if you have a name with more than two of the same letter.
- Teach Mama likes to use letter magnets to spell their names!
- Learn to spell their name while making a rainbow!
Name Spelling Activities
Type on the Computer
Hands On As We Grow
Name Scavenger Hunt
Hands On As We Grow
Play Dough Printed Letters
The Imagination Tree
Cookie Names
Danya Banya
Name Rockets
Ms.
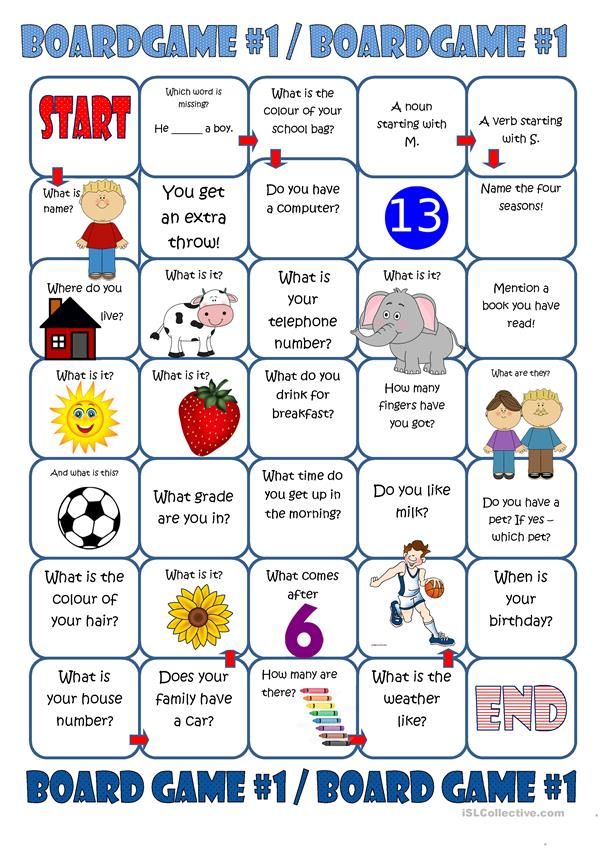
Paint Stick Names
Tip Junkie
Post-It Note Names
Busy Toddler
Name Necklaces
Little Giraffes
DIY Name Cube Game
Toddler Approved
Scrambled Letters Name Game
Kids' Activities
I Spy My Name
Nurture Store
Rock Your Name
Time for Play
Go Fish for Your Name
Kids Activity Zone
Name Stamping
Frugal Fun 4 Boys & Girls
DIY Name Mobile
Cere's Childcare
Shoot Your Name Archery Activity
Toddler Approved
Custom Printable Name Worksheets
Handwriting Worksheets
DIY Name Puzzle
Happy Hooligans
ABC Block Names
Having Fun At Home
Letter Magnet Spelling
Teach Mama
Rainbow Spelling
Hands On As We Grow
Not quite to names yet?
Letter learning comes first.
Find 20+ letter learning activities that are perfect for preschoolers!
SHARE POST
About Jamie Reimer
Jamie learned to be a hands on mom by creating activities, crafts and art projects for her three boys to do. Jamie needed the creative outlet that activities provided to get through the early years of parenting with a smile! Follow Jamie on Pinterest and Instagram!
Reader Interactions
52 Name Recognition Activities for Preschoolers
This post may contain affiliate links, which means that at no cost to you, I may earn a small sum if you click through and make a purchase.by Sarah Punkoney, MAT
Learning name recognition is one of the most important skills taught by preschool teachers. These 52 fun name activities will help preschoolers learn letter recognition, practice name recognition, write letters, and learn to spell their names in fresh new ways all year long!
Below you’ll find a variety of hands-on name recognition activities, printables, crafts, and sensory bins organized by season. This will help you easily find activities to fit with your lesson plans this year.
Fun Name Recognition Activities
My preschooler has recently become obsessed with his name. He’s learning to recognize letters and has been asking me to write his name on strips of paper for him. Then he tapes them on random pieces of furniture as if to claim them as his own.
He’s even realized that he shares a last name with his brother! I credit this realization to the karate cIass they’ve both started taking where they’re addressed by their last name. It’s really cute to watch this awareness of his first and last name develop. These name recognition activities will really help him further develop this important preschool skill as well as fine motor skills.
FAQs About Name Recognition Activities
How do you teach name recognition?
To teach name recognition to preschoolers, you must first start with letter recognition. Preschoolers will learn to recognize the first letter of their name, followed by the shape of their name. Labeling items with their name will help with this.
Once they can recognize the shape of their name, you can encourage them to orally spell it by singing silly name songs and doing hands-on activities.
Finally, you can teach them to write the letters. Incorporating a variety of name recognition activities into your lesson plans will make learning fun and exciting!
How can I help my child recognize their name if they’re struggling?
Stay calm; a solid way to help students who are struggling to recognize their name is to include more repetition in their activities. Label everything with their name and point it out to them daily. Plan a variety of fun activities to help them recognize the individual letters of their name or the shape of their name. You can also sing silly name songs, read books with their name in them, and practice spelling and writing their name.
Should a 3-year-old write their name?
A 3-year-old is not too young to start learning to write their name. Begin by encouraging them to write big letters in a fun way! Using a salt tray or air writing is a great first activity. Just make sure not to push them too hard; you don’t want them to get frustrated and discouraged if this is a challenge for them.
The Best Name Spelling Sheets!
Are your preschoolers struggling to learn how to spell their names?
These Editable Name Spelling Practice Mats will be a game changer for your preschooler. Just type in your preschooler’s names and hit print!
You can even use them in kindergarten!
THESE EDITABLE NAME SPELLING PRACTICE MATS WILL HELP PRESCHOOLERS DEVELOP:- name recognition
- name spelling skills
- one-to-one correspondence in letter sounds
- letter formation skills
- proper pencil grasp
- letter sequencing
- left-to-right progression
This is an editable PDF, which means all you have to do is type in your preschooler’s names on the class list and the sheets will automatically generate!
Get ready to have a blast teaching your students with this collection of creative, easy-to-use activities! You’ll find a variety of hands-on name recognition activities, printables, crafts, and sensory bins organized by season.
Kids will love these 52 learning activities! Name recognition is an essential skill for preschoolers to learn. These ideas will keep kids learning with an assortment of fun activities. They’ll also keep your preschool lesson plans fresh and new. I hope this list of name recognition ideas helps round out your preschoolers education!
Grab Your Name Spelling Sheets!
These name spelling sheets were a game changer for me in my preschool classroom.
Sarah Punkoney, MAT
I’m Sarah, an educator turned stay-at-home-mama of five! I’m the owner and creator of Stay At Home Educator, a website about intentional teaching and purposeful learning in the early childhood years. I’ve taught a range of levels, from preschool to college and a little bit of everything in between. Right now my focus is teaching my children and running a preschool from my home. Credentials include: Bachelors in Art, Masters in Curriculum and Instruction.
stayathomeeducator.com/
NLP.
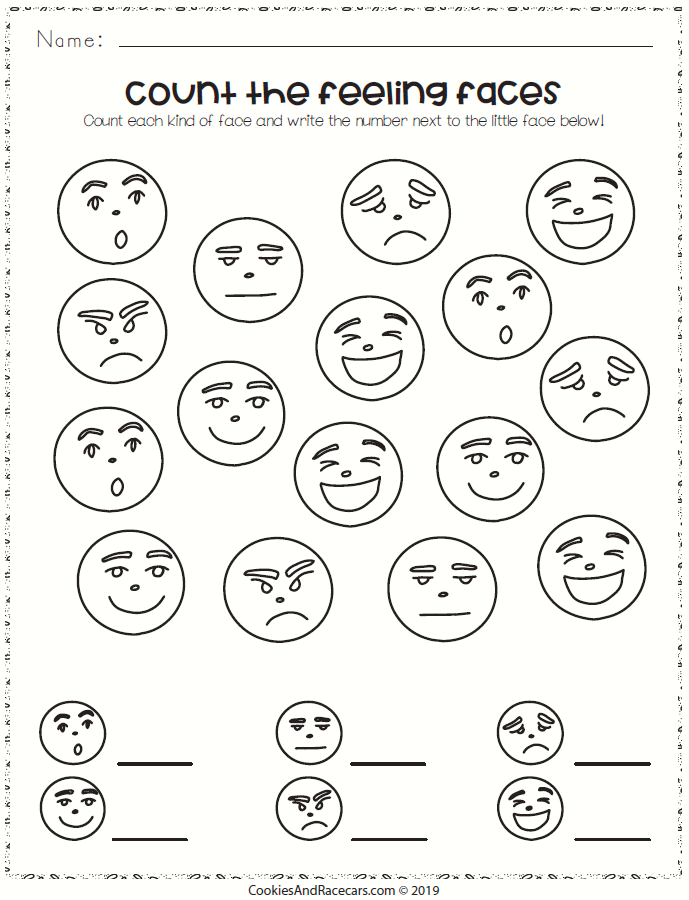
The first part of the article about the basics of NLP can be read here. And today we will talk about one of the most popular NLP tasks - the extraction of named entities (Named-entity recognition, NER) - and analyze in detail the architectures for solving this task.
The task of NER is to highlight spans of entities in the text (span is a continuous piece of text). Suppose there is a news text, and we want to highlight entities in it (some pre-fixed set - for example, persons, locations, organizations, dates, and so on). The task of NER is to understand that the text section “ January 1, 1997 ” is a date, “ Kofi Annan ” is a person, and “ UN ” is an organization.
What are named entities? In the first, classical setting, which was formulated at the MUC-6 conference in 1995, these are persons, locations and organizations. Since then, there have been several available corpora, each with its own set of named entities. Typically, new entity types are added to people, locations, and organizations. The most common of them are numeric (dates, amounts of money), as well as Misc entities (from miscellaneous - other named entities; an example is iPhone 6). nine0003
Why solve the NER problem
It is easy to understand that even if we learn well to highlight persons, locations and organizations in the text, this is unlikely to arouse great interest among customers. Although, of course, the problem in the classical formulation also has some practical application.
One of the scenarios when solving a problem in the classical formulation may still be needed is the structuring of unstructured data. Suppose you have some text (or a set of texts), and the data from it needs to be entered into the database (table). Classic named entities can correspond to the rows of such a table or serve as the content of some cells. Accordingly, in order to correctly fill out the table, you must first highlight in the text the data that you will enter into it (usually after this there is one more stage - the identification of entities in the text, when we understand that the spans are “ UN ” and “ United Nations ” refer to the same organization; however, the task of identification or entity linking is another task, and we will not talk about it in detail in this post).
However, there are several reasons why NER is one of the most popular NLP tasks.
First, the extraction of named entities is a step towards “understanding” the text. This can be of value in its own right, or it can help you better solve other NLP problems. nine0003
So, if we know where entities are highlighted in the text, then we can find text fragments that are important for some task. For example, we can select only those paragraphs where entities of a certain type occur, and then work only with them.
Let's say you receive a letter, and it would be nice to make a snippet of only the part where there is something useful, and not just “ Hello, Ivan Petrovich ”. If you can highlight named entities, the snippet can be made smart by showing the part of the email where there are entities of interest to us (rather than just showing the first sentence of the email, as is often done). Or you can simply highlight the necessary parts of the letter in the text (or, directly, the entities that are important to us) for the convenience of analysts. nine0003
In addition, entities are rigid and reliable collocations, their selection can be important for many problems. Let's say you have the name of a named entity, and whatever it is, most likely, it is continuous, and all actions with it must be performed as a single block. For example, to translate the name of the entity into the name of the entity. You want to translate “ Pyaterochka Store ” into French as a single piece, and not break it into several unrelated fragments. The ability to define collocations is also useful for many other tasks - for example, for syntactic parsing. nine0003
Without solving the NER problem, it is hard to imagine solving many NLP problems, for example, resolving pronominal anaphora or building question-answer systems. The pronominal anaphora allows us to understand which element of the text the pronoun refers to. For example, let's say we want to analyze the text “ Charming galloped on a white horse. The princess ran out to meet him and kissed him .” If we highlighted the Person entity on the word “Charming”, then the machine will be able to understand much more easily that the princess, most likely, did not kiss the horse, but Prince Charming. nine0003
Now let's give an example of how named entity extraction can help in building question-answer systems. If you ask your favorite search engine the question “ Who played the role of Darth Vader in the movie “The Empire Strikes Back” ”, then with a high probability you will get the right answer. This is done just by highlighting named entities: we select entities (film, role, etc.), we understand that we are being asked, and then we look for the answer in the database.
Perhaps the most important consideration that makes the NER problem so popular is that the problem statement is very flexible. In other words, no one forces us to single out exactly locations, persons and organizations. We can select any continuous fragments of text we need, which are somewhat different from the rest of the text. As a result, you can choose your own set of entities for a specific practical task coming from the customer, mark up the corpus of texts with this set, and train the model. This scenario is ubiquitous, and it makes NER one of the most frequently solved NLP problems in the industry. nine0003
I will give a couple of examples of such user cases from specific customers, in the solution of which I had a chance to take part.
Here is the first one: suppose you have a set of invoices (money transfers). Each invoice has a textual description, which contains the necessary information about the transfer (who, to whom, when, what and for what reason sent). For example, company X transferred $10 to company Y on such-and-such date in such-and-such a way for such-and-such. The text is rather formal, but written in a lively language. Banks have specially trained people who read this text and then enter the information contained in it into the database. nine0003
We can select a set of entities that correspond to the columns of a table in the database (company names, transfer amount, transfer date, transfer type, etc.) and learn how to automatically highlight them. After that, it remains only to enter the selected entities into the table, and people who previously read texts and entered information into the database will be able to do more important and useful tasks.
The second use case is as follows: you need to analyze emails with orders from online stores. To do this, you need to know the order number (so that all letters related to this order are marked or put in a separate folder), as well as other useful information - the name of the store, the list of goods that were ordered, the amount on the check, etc. All this - order numbers, store names, etc. - can be considered named entities, and it is also easy to learn to isolate them using the methods that we will now analyze. nine0003
If NER is so useful, why isn't it widely used?
Why has the NER problem not been solved everywhere and why are commercial customers still willing to pay not the smallest amount of money for its solution? It would seem that everything is simple: understand which piece of text to highlight, and select it.
But life is not so easy, there are different difficulties.
The classical complexity that prevents us from living when solving a variety of NLP problems is various kinds of ambiguities in the language. For example, polysemantic words and homonyms (see examples in part 1). There is also a separate type of homonymy that is directly related to the NER task - completely different entities can be called the same word. For example, let's say we have the word " Washington ”. What is it? Person, city, state, store name, dog name, object, something else? To single out this section of the text as a specific entity, a lot must be taken into account - the local context (what the previous text was about), the global context (knowledge of the world). A person takes all this into account, but it is not easy to teach a machine to do this.
The second difficulty is technical, but it should not be underestimated. No matter how you define the entity, most likely, some borderline and difficult cases will arise - when it is necessary to select the entity, when it is not necessary, what to include in the entity span and what not, etc. (of course, if our entity is not something slightly variable, like an email; however, you can usually select such trivial entities using trivial methods - write a regular expression and not think about any machine learning). nine0003
Let's say we want to highlight store names.
In the text “ Welcome to the Professional Metal Detector Store”, we almost certainly want to include the word “shop” in our essence - this is clearly part of the name.
Another example is “ Welcome to Volkhonka Prestige, your favorite brand store at affordable prices ”. Probably, the word “shop” should not be included in the abstract - this is clearly not part of the name, but simply its description. In addition, if you include this word in the title, you must also include the words “- your favorite”, and this, perhaps, is not at all desirable to do. nine0003
Third example: “ Nemo pet store writes to you”. It's not clear if "pet store" is part of the name or not. It seems that in this example, any choice will be adequate. However, it is important that we need to make this choice and fix it in the markup instructions so that such examples are marked up in the same way in all texts (if this is not done, machine learning will inevitably start to make mistakes due to inconsistencies in the markup).
There are many such borderline examples, and if we want the markup to be consistent, all of them must be included in the markup instructions. Even if the examples themselves are simple, you need to take them into account and calculate them, and this will make the instruction larger and more complicated. nine0003
Well, the more complex the instruction, the more qualified markers you need. It’s one thing when a markup needs to determine whether a letter is an order text or not (although there are subtleties and edge cases here too), and another thing when a markup needs to read a 50-page instruction, find specific entities, understand what to include in abstract and what not.
Skilled scribers are expensive and usually slow to work. You will spend the money for sure, but it’s not at all a fact that the markup will be perfect, because if the instructions are complicated, even a qualified person can make a mistake and misunderstand something. To combat this, multiple markups of the same text by different people are used, which further increases the price of markup and the time it takes to prepare it. Avoiding this process or even seriously reducing it will not work: in order to learn, you need to have a high-quality training sample of reasonable size. nine0003
These are the two main reasons why NER has not yet conquered the world and why apple trees still do not grow on Mars.
How to understand whether the problem is well solved NER
I'll tell you a little about the metrics that people use to evaluate the quality of their solution to the NER problem, and about standard cases.
The main metric for our problem is a strict f-measure. Let's explain what it is.
Let us have a test markup (the result of our system operation) and a reference (correct markup of the same texts). Then we can calculate two metrics - accuracy and recall. Accuracy is the proportion of true positive entities (i.e., entities that we selected in the text that are also present in the reference) relative to all entities identified by our system. And completeness is the proportion of true positive entities relative to all entities present in the reference. An example of a very accurate but incomplete classifier is a classifier that picks out one correct object in the text and nothing else. An example of a very complete, but generally inaccurate classifier is a classifier that selects an entity in any segment of the text (thus, in addition to all the reference entities, our classifier selects a huge amount of garbage). nine0003
F-measure is the harmonic mean of precision and recall, a standard metric.
As we discussed in the previous section, creating markup is expensive. Therefore, there are not very many available cases with markup.
For the English language, there is some diversity - there are popular conferences where people compete in solving the NER problem (and markup is created for the competition). Examples of such conferences where their own corpuses with named entities were created are MUC, TAC, CoNLL. All these corpora consist almost exclusively of news texts. nine0003
The main corpus on which the quality of the solution of the NER problem is evaluated is the CoNLL 2003 corpus (here is a link to the corpus itself, here is an article about it). There are approximately 300 thousand tokens and up to 10 thousand entities. Now SOTA systems (state of the art - that is, the best results at the moment) show an f-measure of about 0.93 on this case.
Things are much worse for the Russian language. There is one public corpus (FactRuEval 2016, here is an article about it, here is an article on Habré), and it is very small - there are only 50 thousand tokens. At the same time, the body is quite specific. In particular, the rather controversial entity LocOrg (location in an organizational context) stands out in the corpus, which is confused with both organizations and locations, as a result of which the quality of highlighting the latter is lower than it could be. nine0003
How to solve the NER problem
Reducing the NER problem to a classification problem
Although entities are often verbose, the NER problem is usually reduced to a classification problem at the token level, i.e. each token belongs to one of several possible classes. There are several standard ways to do this, but the most common one is called the BIOS scheme. The scheme is to add some prefix to the entity label (for example, PER for persons or ORG for organizations), which indicates the position of the token in the entity span. More details:
B - from the word beginning - the first token in the entity span that consists of more than 1 word.
I - from the words inside - this is what is in the middle.
E - from the word ending, this is the last token of an entity that consists of more than 1 element.
S - single. We add this prefix if the entity consists of one word.
Thus, we add one of 4 possible prefixes to each entity type. If the token does not belong to any entity, it is marked with a special label, usually denoted OUT or O.
Let's take an example. Suppose we have the text “ Carl Friedrich Hieronymus von Munchausen was born in Bodenwerder ”. There is one wordy entity here - the person "Karl Friedrich Hieronymus von Münghausen" and one one-word entity - the location "Bodenwerdere".
So BIOES is a way to map projections of spans or annotations to the token level.
It is clear that with this markup we can unambiguously set the boundaries of all annotations of entities. Indeed, for each token, we know whether it is true that the entity begins with this token or ends on it, which means whether to end the entity annotation on this token, or extend it to the next tokens. nine0003
The vast majority of researchers use this method (or its variations with fewer labels - BIOE or BIO), but it has several significant drawbacks. The main one is that the schema does not allow working with nested or overlapping entities. For example, the entity “ Moscow State University named after M.V. Lomonosov ” is one organization. But Lomonosov himself is a person, and it would also be nice to set this in the markup. Using the markup method described above, we will never be able to convey both of these facts at the same time (because we can make only one mark for one token). Accordingly, the Lomonosov token can be either part of the annotation of the organization, or part of the annotation of the person, but never both at the same time. nine0003
Another example of nested entities: “ Department of Mathematical Logic and Theory of Algorithms, Faculty of Mechanics and Mathematics of Moscow State University ”. Here, ideally, I would like to highlight 3 nested organizations, but the above markup method allows you to select either 3 non-overlapping entities, or one entity that has the entire fragment as annotated.
In addition to the standard way to reduce a problem to token-level classification, there is also a standard data format that is convenient for storing markup for a NER problem (as well as for many other NLP problems). This format is called CoNLL-U. nine0003
The main idea of the format is as follows: we store data in the form of a table, where one row corresponds to one token, and columns correspond to a specific type of token features (including the word itself — the word form). In a narrow sense, the CoNLL-U format specifies exactly which types of features (i.e. columns) are included in the table - a total of 10 types of features per token. But researchers usually consider the format more broadly and include those types of features that are needed for a specific problem and a method for solving it. nine0003
Below is an example of data in a CoNLL-U-like format, where 6 types of features are considered: the number of the current sentence in the text, word form (i.e. the word itself), lemma (initial form of the word), POS tag (part of speech) , morphological characteristics of the word and, finally, the label of the entity allocated on this token.
How did you solve the NER problem before?
Strictly speaking, the problem can be solved without machine learning - using rule-based systems (in the simplest version - using regular expressions). This seems outdated and inefficient, but you need to understand that if you have a limited and clearly defined subject area and if the entity itself does not have a lot of variability, then the NER problem is solved using rule-based methods quite efficiently and quickly. nine0003
For example, if you need to isolate emails or numeric entities (dates, amounts of money, or phone numbers), regular expressions can get you there faster than trying to solve a problem with machine learning.
However, as soon as language ambiguities of various kinds come into play (we wrote about some of them above), such simple methods cease to work well. Therefore, it makes sense to use them only for limited domains and for simple and clearly separable entities from the rest of the text. nine0003
Despite all of the above, until the end of the 2000s, SOTA showed systems based on classical machine learning methods in academic buildings. Let's take a quick look at how they worked.
Features
Before the advent of embeddings, the main feature of a token was usually a word form - that is, the index of a word in a dictionary. Thus, each token is assigned a Boolean vector of high dimension (dictionary dimension), where there is 1 in place of the word index in the dictionary, and 0 in other places.0003
In addition to the word form, parts of speech (POS tags), morphological features (for languages without rich morphology - for example, English, morphological features have almost no effect), prefixes (i. e., the first few characters of a word) were often used as token features ), suffixes (similarly, the last few characters of the token), the presence of special characters in the token, and the appearance of the token.
In classical formulations, a very important feature of a token is the type of its capitalization, for example:
- “the first letter is large, the rest are small”,
- “all small letters”,
- “all capital letters”,
- or “non-standard capitalization” in general (observed, in particular, for the “iPhone” token).
If a token has a non-standard capitalization, it is highly likely that the token is some kind of entity, and the type of this entity is unlikely to be a person or location.
In addition to all this, gazetteers - dictionaries of entities - were actively used. We know that Petya, Elena, Akaki are names, Ivanov, Rustaveli, von Goethe are surnames, and Mytishchi, Barcelona, Sao Paulo are cities. It is important to note that entity dictionaries by themselves do not solve the problem (“Moscow” can be part of the name of the organization, and “Elena” is part of the location), but they can improve its solution. However, of course, despite the ambiguity, the belonging of a token to a dictionary of entities of a certain type is a very good and significant sign (so significant that usually the results of solving the NER problem are divided into 2 categories - with and without gazetteers). nine0003
If you're interested in how people solved the NER problem when the trees were big, I suggest you check out Nadeau and Sekine (2007), A survey of Named Entity Recognition and Classification. The methods that are described there are, of course, outdated (even if you cannot use neural networks due to performance limitations, you will probably not use HMM, as it is written in the article, but, say, gradient boosting), but look at the description of the features might make sense.
Interesting features include capitalization patterns (summarized pattern in the article above). They can still help with some NLP tasks. So, in 2018, there was a successful attempt to apply capitalization patterns (word shapes) to neural network methods for solving a problem. nine0003
How to solve the NER problem using neural networks?
NLP almost from scratch
The first successful attempt to solve the NER problem using neural networks was made in 2011.
At the time of publication of this article, it showed a SOTA result on the CoNLL 2003 corpus. But you need to understand that the superiority of the model compared to systems based on classical machine learning algorithms was quite insignificant. In the next few years, methods based on classical ML showed results comparable to neural network methods. nine0003
In addition to describing the first successful attempt to solve the NER problem using neural networks, the article describes in detail many points that are left out of the brackets in most works on the topic of NLP. Therefore, despite the fact that the architecture of the neural network described in the article is outdated, it makes sense to read the article. This will help to understand the basic approaches to neural networks used in solving the NER problem (and more broadly, many other NLP problems).
Let's talk more about the architecture of the neural network described in the article. nine0003
The authors introduce two types of architecture, corresponding to two different ways to take into account the context of the token:
- or use a “window” of a given width (window based approach),
- or consider the whole sentence as context (sentence based approach).
In both cases, the features used are embeddings of word forms, as well as some manual features - capitalization, parts of speech, etc. Let's talk more about how they are calculated. nine0003
We received a list of words from our sentence as input: for example, “ The cat sat on the mat ”.
Let there be K different features for one token (for example, such features can be a word form, part of speech, capitalization, whether our token is the first or last in a sentence, etc.). We can consider all these signs as categorical (for example, a word form corresponds to a Boolean vector of the length of the dimension of the dictionary, where 1 is only on the coordinate corresponding to the index of the word in the dictionary). Let be a Boolean vector corresponding to the value of the i-th feature of the j-th token in the sentence. nine0003
It is important to note that in the sentence based approach, in addition to categorical features determined by words, a feature is used - a shift relative to the token whose label we are trying to determine. The value of this feature for token number i will be i-core, where core is the number of the token whose label we are trying to determine at the moment (this feature is also considered categorical, and the vectors for it are calculated in the same way as for the others).
The next step in finding the features of a token is multiplying each by a matrix called Lookup Table (thus, the Boolean vectors “turn” into continuous ones). Recall that each of is a Boolean vector, in which 1 is in one place, and 0 in other places. Thus, when multiplied by , one of the rows in our matrix is selected. This string is the embedding of the corresponding feature of the token. The matrices (where i can take values from 1 to K) are the parameters of our network that we train along with the rest of the layers of the neural network. nine0003
The difference between the method of working with categorical features described in this article and word2vec that appeared later (we talked about how word2vec wordform embeddings are pre-trained in the previous part of our post) is that here the matrices are initialized randomly, and in word2vec matrices are pre-trained on large corpus on the problem of determining a word by context (or context by word).
Thus, for each token, a continuous feature vector is obtained, which is the concatenation of the results of multiplying all possible by . nine0003
Now let's look at how these features are used in the sentence based approach (window based is ideologically simpler). It is important that we will run our architecture separately for each token (i.e. for the sentence “The cat sat on the mat” we will run our network 6 times). The features in each run are collected the same, with the exception of the feature responsible for the position of the token, the label of which we are trying to determine - the core token.
We take the resulting continuous vectors of each token and pass them through a one-dimensional convolution with filters of not very large dimensions: 3-5. The dimension of the filter corresponds to the dimension of the context that the network takes into account at the same time, and the number of channels corresponds to the dimension of the original continuous vectors (the sum of the dimensions of the embeddings of all features). After applying the fold, we get an m by f matrix, where m is the number of ways the filter can be applied to our data (i. e. sentence length minus filter length plus one) and f is the number of filters used. nine0003
As almost always when working with convolutions, after convolution we use pooling — in this case, max pooling (i.e. for each filter we take the maximum of its value in the entire sentence), after which we get a vector of dimension f. Thus, all the information contained in the proposal, which we may need when determining the label of the core token, is compressed into one vector (max pooling was chosen because we are interested not in the information on the average for the proposal, but in the values of features in its most important sections) . This “flattened context” allows us to collect the attributes of our token throughout the sentence and use this information to determine what label the core token should receive. nine0003
Next, we pass the vector through a multilayer perceptron with some activation functions (in the article - HardTanh), and as the last layer we use a fully connected one with softmax of dimension d, where d is the number of possible token labels.
Thus, the convolutional layer allows us to collect the information contained in the filter dimension window, pooling allows us to highlight the most characteristic information in the sentence (by compressing it into one vector), and the softmax layer allows us to determine what label the token number core has. nine0003
CharCNN-BLSTM-CRF
Now let's talk about the CharCNN-BLSTM-CRF architecture, that is, what was SOTA in the period 2016-2018 (in 2018, architectures based on embeddings on language models appeared, after which the NLP world will never be the same; but this saga is not about this). As applied to the NER problem, the architecture was first described in Lample et al (2016) and Ma & Hovy (2016).
The first layers of the network are the same as in the NLP pipeline described in the previous part of our post. nine0003
First, the context-independent attribute of each token in the sentence is calculated. Signs are usually collected from three sources. The first is the word-form embedding of the token, the second is symbolic features, and the third is additional features: information about capitalization, part of speech, etc. The concatenation of all these features is the context-independent feature of the token.
We talked about word-form embeddings in detail in the previous part. We have listed additional features, but we did not say exactly how they are built into the neural network. The answer is simple - for each category of additional features, we learn embedding of not very large size from scratch. These are exactly the Lookup tables from the previous paragraph, and we learn them in exactly the same way as described there. nine0003
Now let's talk about the structure of symbolic features.
Let's first answer the question of what it is. It's simple - for each token we want to receive a vector of features of a constant size, which depends only on the characters that make up the token (and does not depend on the meaning of the token and additional attributes, such as part of speech).
Now let's move on to the description of the CharCNN architecture (as well as the related CharRNN architecture). We are given a token, which consists of some characters. For each character, we will issue a vector of some not very large dimension (for example, 20) - character embedding. Symbolic embeddings can be pre-trained, but most often they learn from scratch - there are a lot of symbols even in a not very large corpus, and symbolic embeddings must be adequately trained. nine0003
So, we have embeddings of all the characters of our token, as well as additional characters that denote the boundaries of the token - paddings (usually padding embeddings are initialized with zeros). Based on these vectors, we would like to obtain one vector of some constant dimension, which is a symbolic attribute of the entire token and reflects the interaction between these symbols.
There are 2 standard ways.
A slightly more popular one is to use one-dimensional convolutions (which is why this part of the architecture is called CharCNN). We do this in exactly the same way as we did with the words in the sentence based approach in the previous architecture. nine0003
So, we pass the embeddings of all characters through convolution with filters of not very large dimensions (for example, 3), we get the dimension vectors of the number of filters. We perform max pooling on these vectors, we get 1 vector of the dimension of the number of filters. It contains information about the symbols of the word and their interaction and will be a vector of symbolic features of the token.
The second way to turn character embeddings into one vector is to feed them into a two-way recurrent neural network (BLSTM or BiGRU; what it is, we described in the first part of our post). Usually, the token's symbolic token is simply the concatenation of the last states of the forward and reverse RNN. nine0003
So, let us be given a context-independent vector of token features. According to it, we want to get a context-sensitive feature.
This is done with BLSTM or BiGRU. At the ith time, the layer outputs a vector that is the concatenation of the corresponding outputs of the forward and reverse RNN. This vector contains information about both the previous tokens in the sentence (it is in the forward RNN) and the next ones (it is in the reverse RNN). Therefore, this vector is a context-sensitive feature of the token. nine0003
This architecture can be used in a wide variety of NLP tasks, so it is considered an important part of the NLP pipeline.
Let us return, however, to the NER problem. Having received the context-dependent features of all tokens, we want to get the correct label for each token. This can be done in many ways.
A simpler and more obvious way is to use a fully connected layer with softmax of dimension d as the last layer, where d is the number of possible token labels. This way we get the probabilities of the token having each of the possible labels (and we can choose the most probable one). nine0003
This method works, but has a significant drawback - the token label is calculated independently of the labels of other tokens. We take into account the neighboring tokens themselves at the expense of BiRNN, but the label of the token depends not only on neighboring tokens, but also on their labels. For example, regardless of tokens, the label I-PER occurs only after B-PER or I-PER.
The standard way to account for interactions between label types is to use CRFs (conditional random fields). We won't go into detail about what it is (there's a good description here), but we'll mention that CRF optimizes the entire label chain, not every element in that label chain. nine0003
So, we have described the CharCNN-BLSTM-CRF architecture, which was SOTA in the NER task before the advent of embeddings on language models in 2018.
In conclusion, let's talk a little about the significance of each element of the architecture. For the English language, CharCNN gives an increase in the f-measure by approximately 1%, CRF - by 1-1. 5%, and additional features of the token do not lead to quality improvement (if you do not use more complex techniques such as multi-task learning, as in the article by Wu et al (2018)). BiRNN is the basis of the architecture, which, however, can be replaced by a transformer. nine0003
We hope that we have been able to give readers some idea of the NER problem. Although this task is important, it is quite simple, which allowed us to describe its solution within the framework of one post.
Ivan Smurov,
Head of NLP Advanced Research Group
NER - Named Entity Recognition
What is NER?
Names of people, names of organizations, books, cities, and other proper names are called "named entities" (named entities), and the task itself is called "recognition of named entities". In English "Named entity recognition" or NER for short; this abbreviation is also regularly used in Russian-language texts. nine0003
In fact, there are two tasks behind one NER task: 1) to detect that some sequence of words is a named entity; 2) understand which class (person's name, organization name, city, etc. ) this named entity belongs to. Each of the stages has its own difficulties.
What are named entities
Let's start with the second step: what classes of named entities you usually want to find. There is no standard set of classes. Almost always, they try to extract the names of people and the names of places and organizations, and then everything depends on the specific tasks that need to be solved, or on the capabilities of the pre-trained system that is planned to be used. Also, the NER task includes extracting dates, amounts of money (number + currency), which do not intuitively look like named entities. nine0003
Many named entities can refer to different classes in different contexts: the word "Pushkin" can be a person, city, club name, etc. Understanding exactly which class a word belongs to in a particular context is a difficult task, which is now a lot of work.
Why is it hard to find named entities?
At first glance, it seems that there should be no particular problems with named entities, most of them are proper names, they are capitalized, so it is not difficult to find them in the text. First, many languages do not have capital letters at all (for example, in Chinese or Arabic). But even in European languages, one has to face difficulties. Main difficulties:
- Named entities are rarely a single word. For example, from the sentence "Dr. Vladimir Bomgard called" you need to extract at least the first and last name - "Vladimir Bomgard", and for many tasks it is useful to be able to find the full "name" of the person about whom it is said: "Dr. Vladimir Bomgard". Similarly, with other named entities: "Ministry of Education of the Russian Federation", "October 19", "100,000 dinars", etc.
- Boundaries are not always obvious, for example, the phrase "Ivan Vasilyevich and partners" may be the name of some organization, or it may mean some Ivan Vasilyevich and his partners separately. nine0168
- Not only proper names are capitalized. This is true for Russian, and even more so for English, but it is especially clear in German, where all nouns are capitalized.
How do you work with named entities?
Although most named entities consist of multiple words, this problem typically considers individual words and decides whether the word is part of the named entity or not. At the same time, the beginning, middle and end of the named entity are distinguished. nine0003
When marking up named entities, it is customary to use the prefix "B" (beginning) for the first word, "E" for the last word, and "I" (intermediate) for all words in between. Sometimes the prefix "S" (single) is also used to denote a named entity consisting of one word.
Thus, the problem is reduced to word classification. Now, to build a classifier, a neural network model is usually trained on a large number of texts with named entity markup. Models are usually based on modules that allow you to take into account the context on both sides of the analyzed word (for example, bi-LSTM or bi-RNN). nine0003
Classical classifiers working with a predefined set of features also give good results.